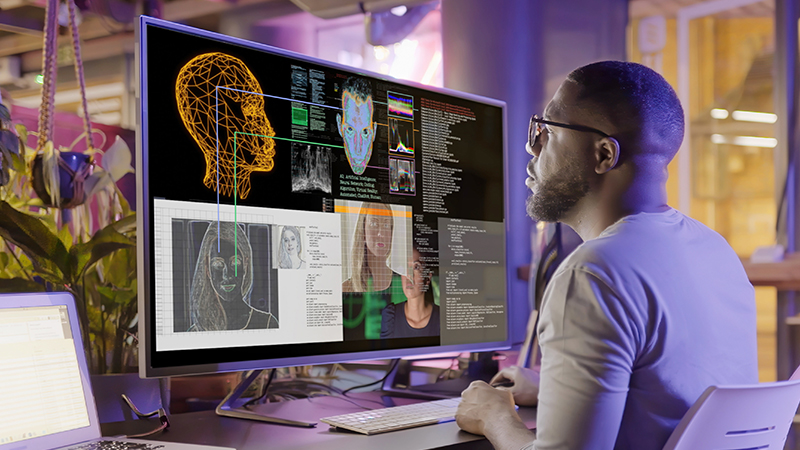
Artificial intelligence moves fast—so fast that predictions about its future can become outdated almost overnight. Back in 2023 and 2024, LIFT Labs made a series of bold AI predictions based on insights garnered from various pilots and POCs with startups from our portfolio. As we worked with our growing portfolio of AI startups, helping them build relationships within Comcast, we gained valuable knowledge about the future of AI, revealing key learnings and implications for enterprises and the market as a whole —but how well did our insights stand the test of time?
Now, in 2025, it’s time to evaluate.
Let’s break down what we got right—and where reality took an unexpected turn.
What We Got Right: AI is a Productivity Powerhouse
LIFT Labs anticipated that AI would revolutionize productivity by automating repetitive tasks and freeing up employees for higher-value, strategic work.
Why This Is Still True in 2025
AI-driven workflow automation is now the norm in major enterprises. AI tools are embedded into daily business operations, slashing time spent on repeatable or mundane tasks.
While AI hasn’t replaced knowledge workers, it has become an essential co-pilot for legal teams, HR departments, marketing functions, finance workflows, and more. Meanwhile, AI agents are growing more sophisticated, mimicking human reasoning and taking on increasingly nuanced tasks. They haven’t achieved full autonomy, but their capabilities are expanding rapidly.
The Takeaway: AI did become a productivity multiplier, just as predicted.
What Else We Got Right: AI is Niche, Not One-Size-Fits-All
We predicted that general-purpose AI wouldn’t be enough for many businesses. Instead, vertical AI solutions—customized for specific industries or functions—would gain traction.
Why This Is Still True in 2025
Businesses want AI that deeply understands their unique business needs. Instead of relying on general-purpose large language models (LLMs), companies are developing task-specific AI models tailored to their needs. The most successful AI companies aren’t building ‘one-size-fits-all’ tools—they’re solving deep, industry-specific problems.
The Takeaway? The real opportunity in AI lies in solving niche business challenges—not creating generic tools.
What We Got Not Quite Right: The Rise of Autonomous AI
We imagined AI making decisions autonomously, operating on behalf of humans, and trained, not only on enterprise data, but on individual preferences, styles, and processes. That hasn’t fully materialized- yet.
What Actually Happened
AI still requires human oversight for critical business decisions due to trust, accuracy, and liability concerns. AI excels at individual tasks but can’t independently handle a person’s entire job description. While AI can mimic human communication styles and suggest data driven decisions, human judgment remains essential—especially for complex business and ethical decisions. One possible reason is that many AI systems must be trained on enormous data sets to properly calibrate, but individuals on their own likely don’t generate the amount of data necessary to train the current iteration of AI systems.
Other substantial hurdles include issues such as accountability for AI-driven decisions and potential biases. Organizations remain cautious, worried about “hallucinations” or errors by LLMs simply making up information when they don’t know the correct answer, or delivering output that isn’t on brand.
Meanwhile, technical limitations still exist as AI systems still struggle with tasks requiring common sense, adaptability, and advanced contextual understanding—areas where human intuition remains superior.
The Takeaway: AI isn’t replacing human judgment—it’s sharpening it.
What We Got Not Quite Right: Enterprises Should Own the Whole Gen AI Stack
Back in 2023, we encouraged enterprises to own their entire Generative AI tech stacks—from data prep and feature engineering to model optimization and evaluation. The thinking? Doing so would safeguard against vendor lock-in, enable niche use case tailoring, and offer more control over model selection and cost structures.
What Actually Happened
Owning the entire stack for every AI use case just isn’t feasible at enterprise scale—nor is it always the smartest strategy. Between speed to market pressures, limited ML engineering bandwidth, and the growing complexity of AI use cases across business units, most companies simply don’t have the time, resources, or organizational alignment to build and maintain bespoke pipelines for everything.
As AI-as-a-Service platforms (like Amazon Bedrock) and AI-powered applications (like Writer, Trullion, Vendict, or Windsurf) get more powerful, the calculus around build vs. buy has continued to evolve. These tools are fast, flexible, and highly specialized—and often outperform custom-built solutions in areas that aren’t core to the business.
The Takeaway: Owning your AI stack isn’t an all-or-nothing decision. The future is hybrid—and smart enterprises are already picking their spots.
Final Verdict: How Did We Do?
We were right about AI’s productivity impact, vertical specialization, and the continued focus on AI security and compliance. We were too optimistic about the speed of enterprise adoption, AI replacing operating systems, and AI achieving full autonomy.
The future of AI is unfolding rapidly, and we’re committed to staying ahead of the curve as we welcome more AI startups into our accelerator programs.